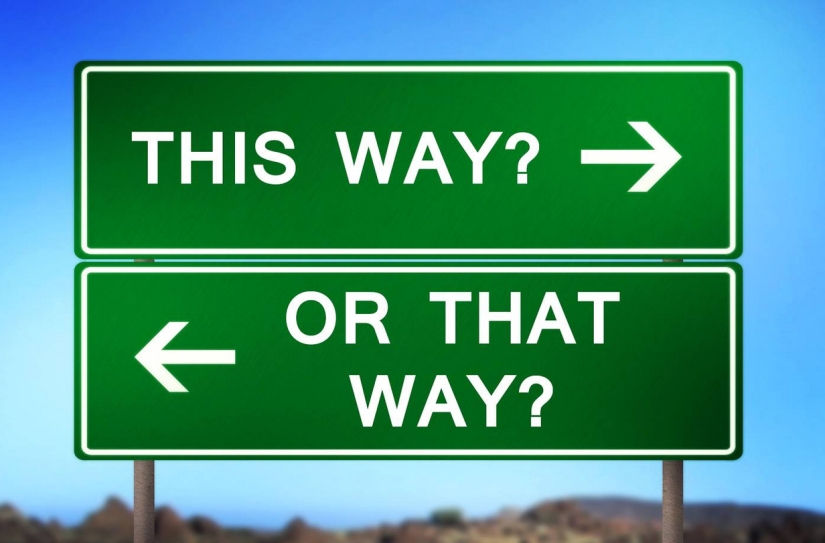
(Picture taken from here)
In making decisions about various issues, policy makers base themselves (hopefully) on detailed analyses of data on the relevant issue in question. Many times the analysis includes tools like summary statistics, or a regression analysis (which is meant to capture the correlation between some variable and another), or general equilibrium models that simulate an economy and households’ behavioral responses to different policies. Which of these tools should the policy maker rely on? What are the strengths and weaknesses of each?
Summary statistics are a raw form of analysis. They simply tell you what the median or average are, for instance. But these statistics carry no information on how one variable affects another or even on what the correlation is between the two variables. So, while these statistics are interesting to look at, they are missing key insights with respect to what should be done.
Regression analysis takes summary statistics a step further and isolates the correlation between two variables of interest (say debt and interest rates, while holding fixed other variables), which makes the connection between the variables clearer. However, regressions have a couple of major issues: first, they do not imply causation. This means that we cannot conclude that if we change one variable it will cause the other one to change as well. And second, even if we were convinced that one variable does cause the other, regressions can only inform the policy maker of the current magnitude of causation. For instance, if we find that one more year of schooling increases earnings by 10%, a policy maker may be compelled to increase enrollment in school by giving subsidies to university students. However, the 10% only holds true for the current situation. If the policy maker increases subsidies and more people enroll in university, it is possible that earnings will decline as more students graduate and increase the supply of labor (which reduces wages). As well, these subsidies come from increased taxation, which means that taxes have to rise. Thus, one must account for the behavioral response of households and businesses to the higher tax. These are all effects that regression analysis (even when done extremely well) does not address well. What I just detailed here is also called “The Lucas Critique“.
General equilibrium models are good workhorses for analyzing all the secondary and ripple effects that regressions cannot account for. These models can anticipate how the increase in the labor supply will affect wages and how higher taxes will affect households and business and what all these effects combined will imply for the economy as a whole. However, these models also have shortcomings. All the secondary effects they estimate depend on the assumptions of the model. Whether these assumptions are realistic enough or not is hard to measure. One way to measure it is to look at how the model performs on dimensions that are not targeted in the calibration of the model (this is a bit technical – think about it as similar to testing the model against data it has never seen before) . Nevertheless, even models that perform well on non-targeted statistics may be wrong, and so even this is not conclusive evidence that the model is “good”. Furthermore, even when these general equilibrium models are done well and are convincing, it usually takes a substantial amount of time to write them, code them, and solve them on the computer. This means that the conclusions drawn from them are not always very timely.
So what should the policy maker rely on? From a “pure economic perspective”, if the policy is of a small magnitude with tight time frames and it is believed that the policy is unlikely to ripple through the economy, then relying on regression analysis would probably do the job. However, if the policy change is substantial and is likely to affect the rest of the economy in meaningful ways, then relying on general equilibrium models is a superior choice as they try to account for those secondary effects as much as possible and using their results may reduce uncertainties and risks for the policy maker.
*Disclaimer: This post was written by Shahar Rotberg on his own time and capacity, and the views expressed herein do not represent the views and opinions of the Canada Mortgage and Housing Corporation.